Russian Scientists Reconstruct Dynamics of Brain Neuron Model Using Neural Network
Researchers from HSE University in Nizhny Novgorod have shown that a neural network can reconstruct the dynamics of a brain neuron model using just a single set of measurements, such as recordings of its electrical activity. The developed neural network was trained to reconstruct the system's full dynamics and predict its behaviour under changing conditions. This method enables the investigation of complex biological processes, even when not all necessary measurements are available. The study has been published in Chaos, Solitons & Fractals.
Neurons are cells that enable the brain to process information and transmit signals. They communicate through electrical impulses, which either activate neighbouring neurons or slow them down. Each neuron has a membrane that allows charged particles, known as ions, to pass through channels in the membrane, generating electrical impulses.
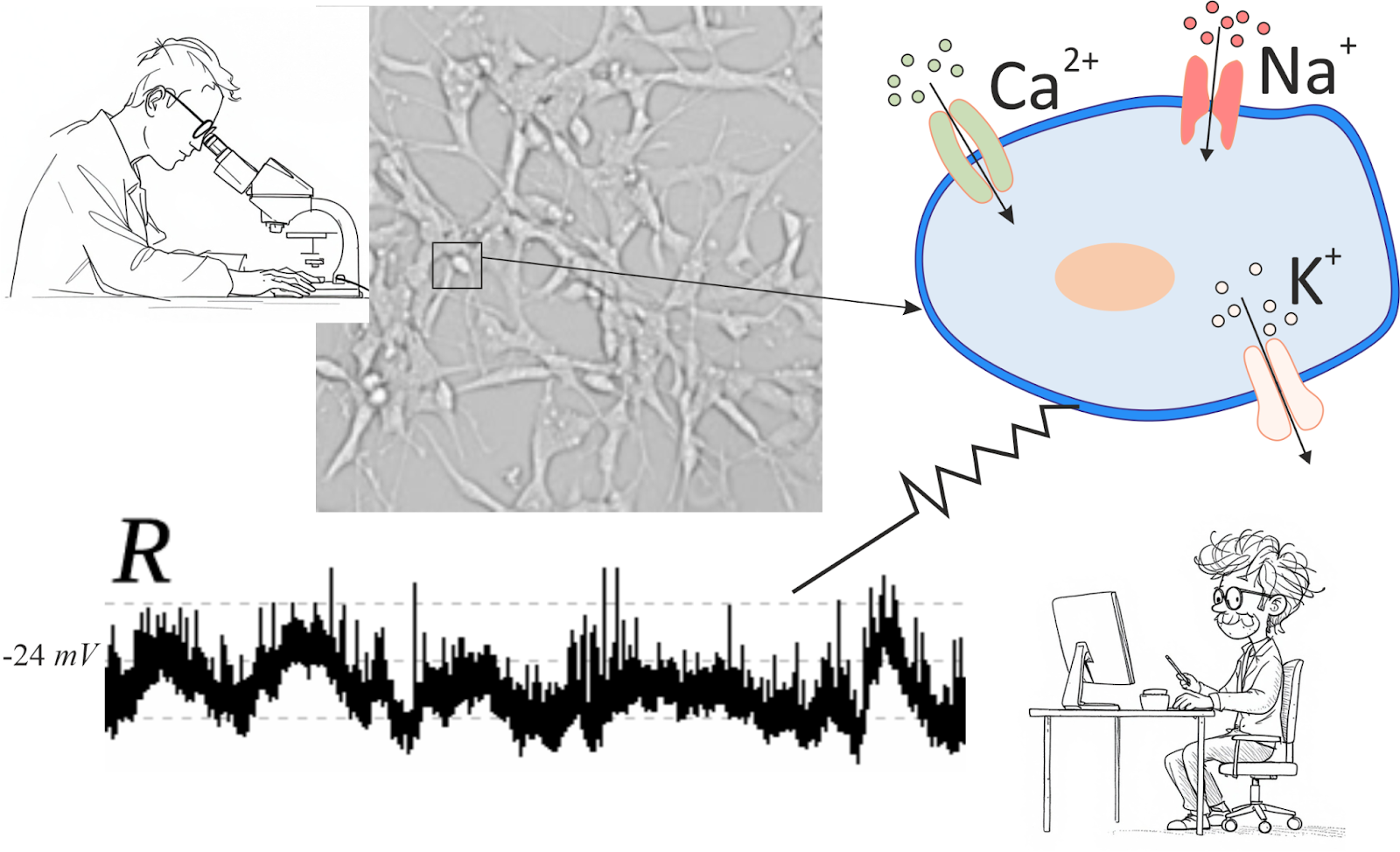
Mathematical models are used to study the function of neurons. These models are often based on the Hodgkin-Huxley approach, which allows for the construction of relatively simple models but requires a large number of parameters and calculations. To predict a neuron's behaviour, several parameters and characteristics are typically measured, including membrane voltage, ion currents, and the state of the cell channels. Researchers from HSE University and the Saratov Branch of the Kotelnikov Institute of Radioengineering and Electronics of the Russian Academy of Sciences have demonstrated the possibility of considering changes in a single control parameter—the neuron's membrane electrical potential—and using a neural network to reconstruct the missing data.
The proposed method consisted of two steps. First, changes in a neuron's potential over time were analysed. This data was then fed into a neural network—a variational autoencoder—that identified key patterns, discarded irrelevant information, and generated a set of characteristics describing the neuron's state. Second, a different type of neural network—neural network mapping—used these characteristics to predict the neuron's future behaviour. The neural network effectively took on the functions of a Hodgkin-Huxley model, but instead of relying on complex equations, it was trained on the data.
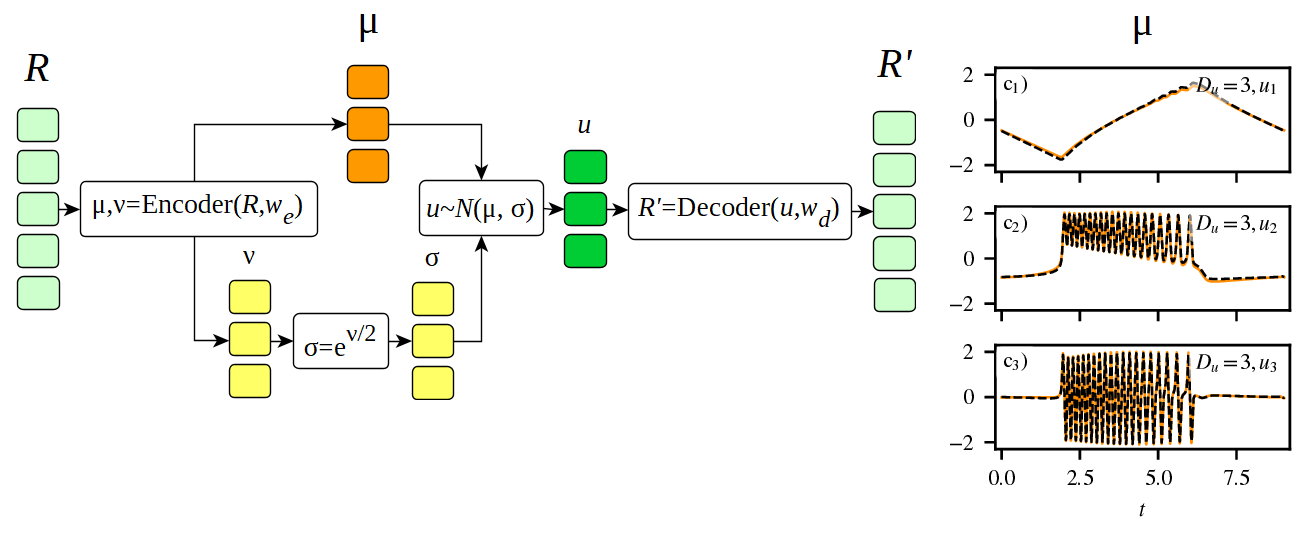
'With the advancement of mathematical and computational methods, traditional approaches are being revisited, which not only helps improve them but can also lead to new discoveries. Models reconstructed from data are typically based on low-order polynomial equations, such as the 4th or 5th order. These models have limited nonlinearity, meaning they cannot describe highly complex dependencies without increasing the error,' explains Pavel Kuptsov, Leading Research Fellow at the Faculty of Informatics, Mathematics, and Computer Science of HSE University in Nizhny Novgorod. 'The new method uses neural networks in place of polynomials. Their nonlinearity is governed by sigmoids, smooth functions ranging from 0 to 1, which correspond to polynomial equations (Taylor series) of infinite order. This makes the modelling process more flexible and accurate.’
Typically, a complete set of parameters is required to simulate a complex system, but obtaining this in real-world conditions can be challenging. In experiments, especially in biology and medicine, data is often incomplete or noisy. The scientists demonstrated by their approach that using a neural network makes it possible to reconstruct missing values and predict the system's behaviour, even with a limited amount of data.
'We take just one row of data, a single example of behaviour, train a model on it, and incorporate a control parameter into it. Imagine it as a rotating switch that can be turned to observe different behaviours. After training, if we start adjusting the switch—ie, changing this parameter—we will observe that the model reproduces various types of behaviours that are characteristic of the original system,' explains Pavel Kuptsov.
During the simulation, the neural network not only replicated the system modes it was trained on but also identified new ones. One of these involves the transition from a series of frequent pulses to single bursts. Such transitions occur when the parameters change, yet the neural network detected them independently, without having seen such examples in the data it was trained on. This means that the neural network does not just memorise examples; it actually recognises hidden patterns.
'It is important that the neural network can identify new patterns in the data,’ says Natalya Stankevich, Leading Research Fellow at the Faculty of Informatics, Mathematics, and Computer Science of HSE University in Nizhny Novgorod. 'It identifies connections that are not explicitly represented in the training sample and draws conclusions about the system's behaviour under new conditions.'
The neural network is currently operating on computer-generated data. In the future, the researchers plan to apply it to real experimental data. This opens up opportunities for studying complex dynamic processes where it is impossible to anticipate all potential scenarios in advance.
The study was carried out as part of HSE University's Mirror Laboratories project and supported by a grant from the Russian Science Foundation.
See also:
Children with Autism Process Sounds Differently
For the first time, an international team of researchers—including scientists from the HSE Centre for Language and Brain—combined magnetoencephalography and morphometric analysis in a single experiment to study children with Autism Spectrum Disorder (ASD). The study found that children with autism have more difficulty filtering and processing sounds, particularly in the brain region typically responsible for language comprehension. The study has been published in Cerebral Cortex.
HSE Scientists Discover Method to Convert CO₂ into Fuel Without Expensive Reagents
Researchers at HSE MIEM, in collaboration with Chinese scientists, have developed a catalyst that efficiently converts CO₂ into formic acid. Thanks to carbon coating, it remains stable in acidic environments and functions with minimal potassium, contrary to previous beliefs that high concentrations were necessary. This could lower the cost of CO₂ processing and simplify its industrial application—eg in producing fuel for environmentally friendly transportation. The study has been published in Nature Communications.
HSE Scientists Reveal How Staying at Alma Mater Can Affect Early-Career Researchers
Many early-career scientists continue their academic careers at the same university where they studied, a practice known as academic inbreeding. A researcher at the HSE Institute of Education analysed the impact of academic inbreeding on publication activity in the natural sciences and mathematics. The study found that the impact is ambiguous and depends on various factors, including the university's geographical location, its financial resources, and the state of the regional academic employment market. A paper with the study findings has been published in Research Policy.
Group and Shuffle: Researchers at HSE University and AIRI Accelerate Neural Network Fine-Tuning
Researchers at HSE University and the AIRI Institute have proposed a method for quickly fine-tuning neural networks. Their approach involves processing data in groups and then optimally shuffling these groups to improve their interactions. The method outperforms alternatives in image generation and analysis, as well as in fine-tuning text models, all while requiring less memory and training time. The results have been presented at the NeurIPS 2024 Conference.
When Thoughts Become Movement: How Brain–Computer Interfaces Are Transforming Medicine and Daily Life
At the dawn of the 21st century, humans are increasingly becoming not just observers, but active participants in the technological revolution. Among the breakthroughs with the potential to change the lives of millions, brain–computer interfaces (BCIs)—systems that connect the brain to external devices—hold a special place. These technologies were the focal point of the spring International School ‘A New Generation of Neurointerfaces,’ which took place at HSE University.
New Clustering Method Simplifies Analysis of Large Data Sets
Researchers from HSE University and the Institute of Control Sciences of the Russian Academy of Sciences have proposed a new method of data analysis: tunnel clustering. It allows for the rapid identification of groups of similar objects and requires fewer computational resources than traditional methods. Depending on the data configuration, the algorithm can operate dozens of times faster than its counterparts. Thestudy was published in the journal Doklady Rossijskoj Akademii Nauk. Mathematika, Informatika, Processy Upravlenia.
Researchers from HSE University in Perm Teach AI to Analyse Figure Skating
Researchers from HSE University in Perm have developed NeuroSkate, a neural network that identifies the movements of skaters on video and determines the correctness of the elements performed. The algorithm has already demonstrated success with the basic elements, and further development of the model will improve its accuracy in identifying complex jumps.
Script Differences Hinder Language Switching in Bilinguals
Researchers at the HSE Centre for Language and Brain used eye-tracking to examine how bilinguals switch between languages in response to context shifts. Script differences were found to slow down this process. When letters appear unfamiliar—such as the Latin alphabet in a Russian-language text—the brain does not immediately switch to the other language, even when the person is aware they are in a bilingual setting. The article has been published in Bilingualism: Language and Cognition.
HSE Experts Highlight Factors Influencing EV Market Growth
According to estimates from HSE University, Moscow leads in the number of charging stations for electric vehicles in Russia, while Nizhny Novgorod ranks first in terms of charging station coverage, with 11.23 electric vehicles per charging station, compared to 14.41 in Moscow. The lack of charging infrastructure is one of the key factors limiting the growth of the electric vehicle market. This is stated in the study titled ‘Socio-Economic Aspects of Introducing Electric Vehicles in Commercial Transportation’ conducted by experts from the Institute of Transport Economics and Transport Policy Studies at HSE University.
Machine Learning Links Two New Genes to Ischemic Stroke
A team of scientists from HSE University and the Kurchatov Institute used machine learning methods to investigate genetic predisposition to stroke. Their analysis of the genomes of over 5,000 people identified 131 genes linked to the risk of ischemic stroke. For two of these genes, the association was found for the first time. The paper has been published in PeerJ Computer Science.